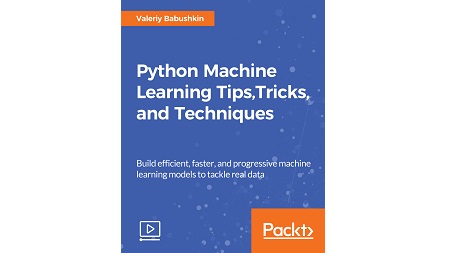
English | MP4 | AVC 1920×1080 | AAC 48KHz 2ch | 2h 46m | 758 MB
Transform your simple machine learning model into a cutting edge powerful version
Machine learning allows us to interpret data structures and fit that data into models to identify patterns and make predictions. Python makes this easier with its huge set of libraries that can be easily used for machine learning. In this course, you will learn from a top Kaggle master to upgrade your Python skills with the latest advancements in Python.
It is essential to keep upgrading your machine learning skills as there are immense advancements taking place every day. In this course, you will get hands-on experience of solving real problems by implementing cutting-edge techniques to significantly boost your Python Machine Learning skills and, as a consequence, achieve optimized results in almost any project you are working on.
Each technique we cover is itself enough to improve your results. However; combining them together is where the real magic is. Throughout the course, you will work on real datasets to increase your expertise and keep adding new tools to your machine learning toolbox.
By the end of this course, you will know various tips, tricks, and techniques to upgrade your machine learning algorithms to reduce common problems, all the while building efficient machine learning models.
We practice real datasets from different fields, progressively increasing our expertise and putting new tools at our disposal. With a combination of these tools, almost any machine learning problem can be solved much faster and with far better overall results.
What You Will Learn
- Tips and tricks to speed up your modeling process and obtain better results
- Make predictions using advanced regression analysis with Python
- Modern techniques for solving supervised learning problems
- Various ways to use ensemble learning with Python to derive optimum results
- Build your own recommendation engine and perform collaborative filtering
- Give your production machine learning system improved reliability
Table of Contents
The Course Overview
Using Feature Scaling to Standardize Data
Implementing Feature Engineering with Logistic Regression
Extracting Data with Feature Selection and Interaction
Combining All Together
Build Model Based on Real-World Problems
Support Vector Machines
Implementing kNN on the Data Set
Decision Tree as Predictive Model
Tricks with Dimensionality Reduction
Combining All Together
Random Forest for Classification
Gradient Boosting Trees and Bayes Optimization
CatBoost to Handle Categorical Data
Implement Blending
Implement Stacking
Memory-Based Collaborative Filtering
Item-to-Item Recommendation with kNN
Applying Matrix Factorization on Datasets
Wordbatch for Real-World Problem
Validation Dataset Tuning
Regularizing Model to Avoid Overfitting
Adversarial Validation
Perform Metric Selection on Real Data
Resolve the captcha to access the links!