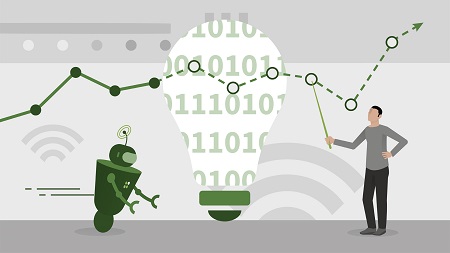
English | MP4 | AVC 1280×720 | AAC 48KHz 2ch | 1h 21m | 165 MB
Building world-class predictive analytics solutions requires recognizing that the challenges of scale and sample size fluctuate greatly at different stages of a project. How do you know how much data to use? What is too little, what is too much? How does your infrastructure need to scale with the volume and demands of the project? This course walks step by step through the strategic and tactical aspects of determining how much data is needed to build an effective predictive modeling solution based on machine learning and what volumes of data are so large that they will create challenges. Instructor Keith McCormick reviews each stage—data selection, data preparation, modeling, scoring, and deployment—with scalability in mind, providing IT professionals, data scientists, and leadership with new insights, perspectives, and collaboration tools.
Note: This course is software agnostic. The emphasis is on strategy and planning. Examples, calculations, and software results shown are for training purposes only.
Topics include:
- Evaluating the proper amount of data
- Assessing data quality and quantity
- Seasonality and time alignment
- Data preparation challenges
- Data modeling challenges
- Scoring machine-learning models
- Deploying models and adjusting data prep and scoring
- Monitoring and maintenance
Table of Contents
1 Scaling machine learning initiatives
2 Defining terms
3 Data and supervised machine learning
4 The nine big data bottlenecks
5 The stages of predictive analytics data
6 Why you might have too little data
7 How much data do I need
8 Balancing
9 Who truly has big data
10 Assessing data
11 Selecting Data that should be left out
12 Seasonality and time alignment
13 Data and the data scientist
14 Aggregate and restructure
15 Dummy coding
16 Feature engineering
17 Understanding the modeling process
18 Slow algorithms Brute force
19 Slow algorithms More calculations
20 Slow algorithms More models
21 How to sample properly
22 Modeling with missing data
23 Scoring traditional ML models
24 Scoring a black box model
25 Scoring an ensemble
26 Batch vs. real-time scoring
27 Data prep and scoring
28 Combining batch and real-time scoring
29 What is model monitoring
30 How often should you rebuild
31 Next steps
Resolve the captcha to access the links!