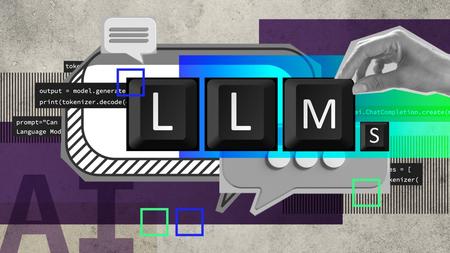
English | MP4 | AVC 1280×720 | AAC 48KHz 2ch | 1h 21m | 153 MB
As large language models are becoming increasingly prevalent in various industries, professionals need to understand how to build, fine-tune, and deploy these models effectively and responsibly. In this course, learn the foundations of building, fine-tuning, and deploying LLMs in real-world applications. Instructors Soham Chatterjee and Archana Vaidheeswaran start with an introduction to LLMs and their evolution in the AI landscape. They then dive into LLM architectures, show you how to fine tune strategies for custom tasks, explain why and how to compress LLMs, and, finally, cover important aspects of prompt engineering. Through the course, they offer a series of challenges and solutions so you can practice the lessons as you go.
Table of Contents
Introduction
1 LLMs for industry
2 Industry-specific LLMs
Introduction to LLMs and Their Applications
3 Understanding LLMs and their evolution
4 Real-world applications of LLMs
Diving Into LLM Architectures
5 Overview of LLM architectures
6 How LLMs process and generate text
7 The building blocks of LLMs
8 Using a simple LLM
9 Challenge LLM for sentiment analysis
10 Solution LLM for sentiment analysis
Fine-Tuning Strategies for Custom Tasks
11 Introduction to fine-tuning for LLMs
12 Step-by-step guide to fine-tuning LLMs
13 Best practices for fine-tuning LLMs
14 Challenge Fine-tune a pre-trained LLM
15 Solution Fine-tune a pre-trained LLM
Compression Techniques for LLMs
16 Why compress LLMs
17 Introduction to quantization and pruning
18 Hands-on Implementing compression in LLMs
19 Challenge Quantize a LLM
20 Solution Quantize a LLM
Prompt Engineering for Effective LLM Communication
21 What is prompt engineering
22 Best practices for effective prompt engineering
23 Types of prompt engineering
24 Challenge Prompting LLMs to generate text
25 Solution Prompting LLMs to generate text
Conclusion
26 Next steps
Resolve the captcha to access the links!