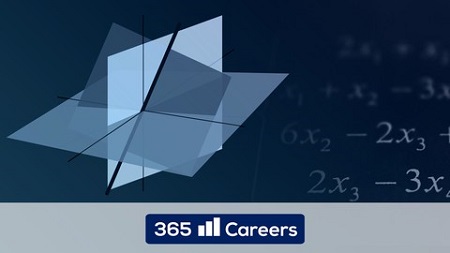
English | MP4 | AVC 1280×720 | AAC 44KHz 2ch | 29 lectures (2h 46m) | 1.24 GB
Acquire the Theoretical and Practical Foundations That Would Allow You to Learn Machine Learning With Understanding
Do you want to learn linear algebra?
You have come to the right place!
First and foremost, we want to congratulate you because you have realized the importance of obtaining this skill. Whether you want to pursue a career in data science, machine learning, data analysis, software engineering, or statistics, you will need to know how to apply linear algebra.
This course will allow you to become a professional who understands the math on which algorithms are built, rather than someone who applies them blindly without knowing what happens behind the scenes.
But let’s answer a pressing question you probably have at this point:
“What can I expect from this course and how it will help my professional development?”
In brief, we will provide you with the theoretical and practical foundations for two fundamental parts of data science and statistical analysis – linear algebra and dimensionality reduction.
Linear algebra is often overlooked in data science courses, despite being of paramount importance. Most instructors tend to focus on the practical application of specific frameworks rather than starting with the fundamentals, which leaves you with knowledge gaps and a lack of full understanding. In this course, we give you an opportunity to build a strong foundation that would allow you to grasp complex ML and AI topics.
The course starts by introducing basic algebra notions such as vectors, matrices, identity matrices, the linear span of vectors, and more. We’ll use them to solve practical linear equations, determine linear independence of a random set of vectors, and calculate eigenvectors and eigenvalues, all preparing you for the second part of our learning journey – dimensionality reduction.
The concept of dimensionality reduction is crucial in data science, statistical analysis, and machine learning. This isn’t surprising, as the ability to determine the important features in a dataset is essential – especially in today’s data-driven age when one must be able to work with very large datasets.
Imagine you have hundreds or even thousands of attributes in your data. Working with such complex information could lead to a variety of problems – slow training time, the possibility of multicollinearity, the curse of dimensionality, or even overfitting the training data.
Dimensionality reduction can help you avoid all these issues, by selecting the parts of the data which actually carry important information and disregarding the less impactful ones.
In this course, we’ll discuss two staple techniques for dimensionality reduction – Principal Components Analysis (PCA), and Linear Discriminant Analysis (LDA). These methods transform the data you work with and create new features that carry most of the variance related to a given dataset. First, you will learn the theory behind PCA and LDA. Then, going through two complete examples in Python, you will see how data transformation occurs in practice. For this purpose, you will get one step-by-step application of PCA and one of LDA. Finally, we will compare the two algorithms in terms of speed and accuracy.
We’ve put a lot of effort to make this course the perfect foundational training for anyone who wants to become a data analyst, data scientist, or machine learning engineer.
What you’ll learn
- Understand the math behind machine learning models
- Become familiar with basic and advanced linear algebra notions
- Be able to solve linear equations
- Determine independency of a set of vectors
- Calculate eigenvalues and eigenvectors
- Perform Linear Discriminant Analysis
- Perform Dimensionality Reduction in Python
- Carry out Principal Components Analysis
- Compare the performance of PCA and LDA for classification with SVMs
Table of Contents
Linear Algebra Essentials
1 What Does The Course Cover
2 Why Linear Algebra
3 Vectors
4 Matrices
5 The Transpose of Vectors and Matrices, the Identity Matrix
6 Linear Independence and Linear Span of Vectors
7 Basis of a Vector Space, Determinant of a Matrix, Inverse of a Matrix
8 Solving Equations of the Form Ax=b
9 The Gauss Method
10 Other Solutions to the Equation Ax=b
11 Determining Linear Independence of a Random Set of Vectors
12 Eigenvalues and Eigenvectors
13 Calculating Eigenvalues
14 Calculating Eigenvectors
Principal Component Analysis (PCA)
15 An Overview of PCA
16 A Step-by-Step Explanation of PCA on California Estates – Example
17 The Theory Behind PCA
18 PCA Covariance Matrix in Jupyter – Analysis and Interpretation
Linear Discriminant Analysis (LDA)
19 Overall Mean and Class Means
20 An Overview of LDA
21 LDA Calculating the Within- and Between-Class Scatter Matrices
22 A Step-by-Step Еxplanation of LDA on a Wine Quality Dataset – Example
23 Calculating the Within- and Between-Class Scatter Matrices
24 Calculating Eigenvectors and Eigenvalues for the LDA
25 Analysis of LDA
26 LDA vs. PCA
27 Setting Up the Classifier to Compare LDA and PCA
28 Coding the Classifier for LDA and PCA
29 Analysis of the Training and Testing Times for the Classifier and Its Accuracy
Resolve the captcha to access the links!