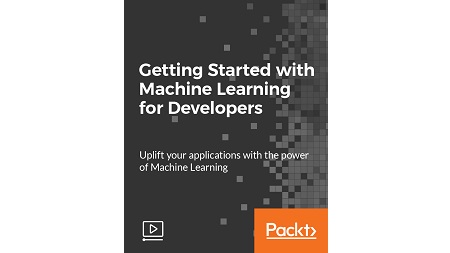
English | MP4 | AVC 1920×1080 | AAC 48KHz 2ch | 1 Hour | 251 MB
Learn the basics of Machine Learning to make high performing day-to-day apps
Most of us have heard about the term Machine Learning, but surprisingly the question frequently asked by developers across the Globe is, “How do I get started in Machine Learning?” One reason could be the vastness of the subject area because people often get overwhelmed by the abstractness of ML and terms such as regression, supervised learning, probability density function, and so on. This systematic guide will teach you various Machine Learning techniques.
You start with the very basics of data and mathematical models in easy-to-follow language that you are familiar with; you will feel at home while implementing the examples. The course introduces you to various libraries and frameworks used in the world of Machine Learning, and then, without wasting any time, you will get to the point and implement regression, clustering, classification, and more, all with fun examples.
This course gives you a glimpse into Machine Learning models and the application of models at scale using clustering, classification, regression, and reinforcement learning, all with fun examples. Hands-on examples will be presented to understand the power of problem-solving with Machine Learning and advanced architectures, software installation, and configuration.
What You Will Learn
- Learn the math and mechanics of Machine Learning
- Understand the steps in the workflow of a Machine Learning process
- Learn K-means clustering
- Learn K-NN clustering
- Explore supervised learning algorithms
Table of Contents
Machine Learning and Statistical Science
1 The Course Overview
2 What is Machine Learning
3 Statistics
4 Probability and Random Variables
5 Differential Calculus Elements
The Learning Process
6 Dataset Retrieval
7 Feature Engineering
8 Dataset Preprocessing
9 Models
Clustering
10 K-Means Algorithm
11 K-NN Algorithm
Linear and Logistic Regression
12 Regression Analysis
13 Linear Regression
14 Data Exploration and Linear Regression in Practice
15 Linear Regression with Gradient Descent
16 Logistic Regression
Resolve the captcha to access the links!